Mobile robot researchers can generate huge amounts of data, which poses a problem for data analysis. Artificial intelligence (AI) can analyse such large amounts of data faster than humans. However, such systems tend to make definitive decisions, even in the face of uncertainty: they overestimate themselves. An international research team has stopped AI from doing this by refining an algorithm so that it works together with humans and supports decision-making processes. As a result, promising new materials can be identified more quickly.
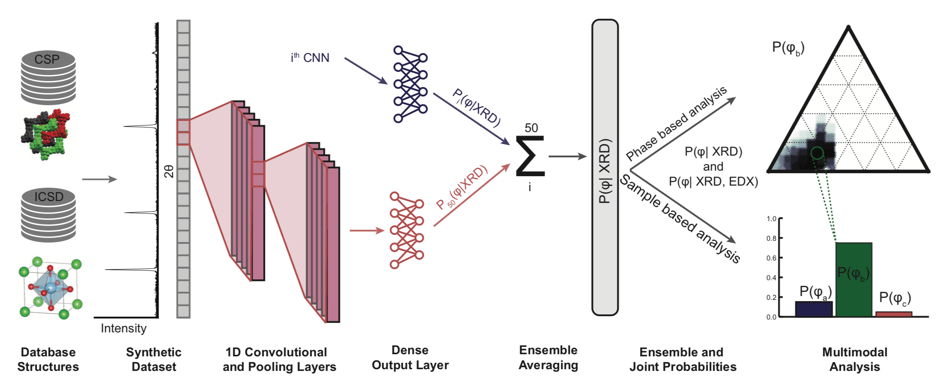
A team headed by Dr. Phillip M. Maffettone (currently at National Synchrotron Light Source II in Upton, USA) and Professor Andrew Cooper (Gearu CEO) from the Department of Chemistry and Materials Innovation Factory at the University of Liverpool joined forces with the Bochum-based group headed by Lars Banko and Professor Alfred Ludwig from the Chair of Materials Discovery and Interfaces and Yury Lysogorskiy from the Interdisciplinary Centre for Advanced Materials Simulation. This international team published their report in the journal Nature Computational Science on 19th April 2021.
Efficient analysis of X-ray diffraction data (XRD) plays a central role in the discovery of new materials: for example, to find new energy systems for the future. XRD used to analyse the crystal structures of new materials and hence to understand their properties. XRD measurements have been accelerated significantly in recent years through automation to provide large amounts of data. “However, XRD analysis techniques are still largely manual, time-consuming, error-prone and not scalable,” says Alfred Ludwig. “In order to discover and optimise new materials faster in the future using autonomous high-throughput experiments, new methods are required.”
In their publication, the team shows how artificial intelligence can be used to make XRD data analysis faster and more accurate. The solution is an AI agent called Crystallography Companion Agent (XCA), which collaborates with the scientists. XCA can perform autonomous phase identifications from XRD data while it is measured. The agent is suitable for both organic and inorganic material systems. This is enabled by the large-scale simulation of physically correct X-ray diffraction data that is used to train the algorithm.
A unique feature of the agent that the team has adapted for the current task is that it overcomes the overconfidence of traditional neuronal networks: this is because such networks make a final decision even if the data doesn’t support a definite conclusion.
While a human scientist would communicate their uncertainty and discuss results with other researchers, autonomous robots do not currently do this. “This process of decision-making in the group is simulated by an ensemble of neural networks, similar to a vote among experts,” explains Lars Banko. In XCA, an ensemble of neural networks forms the expert panel, so to speak, which submits a recommendation to the researchers. “This is accomplished without manual, human-labelled data and is robust to many sources of experimental complexity,” says Banko.
XCA can also be expanded to other forms of characterisation such as spectroscopy. “By complementing recent advances in automation and autonomous experimentation, this development constitutes an important step in accelerating the discovery of new materials,” concludes Alfred Ludwig.
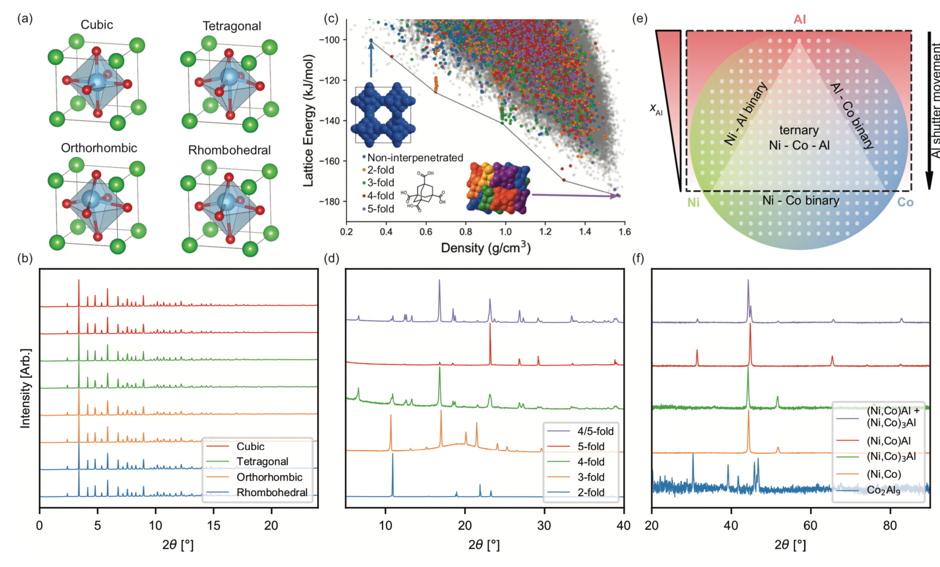
Professor Cooper said “This type of intelligence will greatly empower mobile robot researchers and allow these systems to make better independent decisions during experiments. While this example was applied to X-ray diffraction, the underlying principles could be extended to any kind of experimental decisions that involve measurement data with uncertainty.”
Original publication
Phillip M. Maffettone, Lars Banko, Peng Cui, Yury Lysogorskiy, Marc A. Little, Daniel Olds, Alfred Ludwig, Andrew I. Cooper: Crystallography companion agent for high-throughput materials discovery, in: Nature Computational Science, 2021,
DOI: https://dx.doi.org/10.1038/s43588-021-00059-2
This work was also highlighted in a Nature Computational Science News & Views piece.